We help everyone in the aviation industry get their aircraft more uptime. The key is having a better technology platform to manage everything from maintenance to manuals.
Who we serve
You've got to get more uptime. And that means three things:
No more waiting.
Get everything you need right at your fingertips, including real-time visibility of maintenance data, operations data, regulatory data, and more.
No more wondering.
With an intuitive platform interface that makes it easier to find what you’re looking for, plus a team of experts with deep aviation knowledge.
No more wasted effort.
With an intelligent database that leverages AI and machine learning to simplify everything from documentation to troubleshooting.
After nearly 50 years in the business, we have built a growing reputation for getting our customers more aircraft uptime.
0
Customers
0
Users
0
Aircraft
We are Veryon. New look, same company.
While our dedication to innovation and service will never change as we grow, we felt it was time for a new company name to carry us into the future. Through a creative and strategic process, we honed in on a name that felt equal parts precise, reliable, and visionary.
Browse some of our success stories
Trusted by 7,500+ customers
Find out why thousands of aircraft operators, 25% of the worldwide commercial fleet, and over 100 OEMs all rely on Veryon.
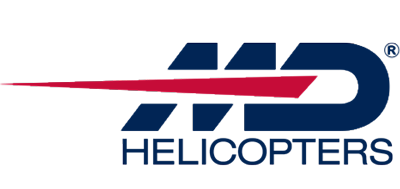
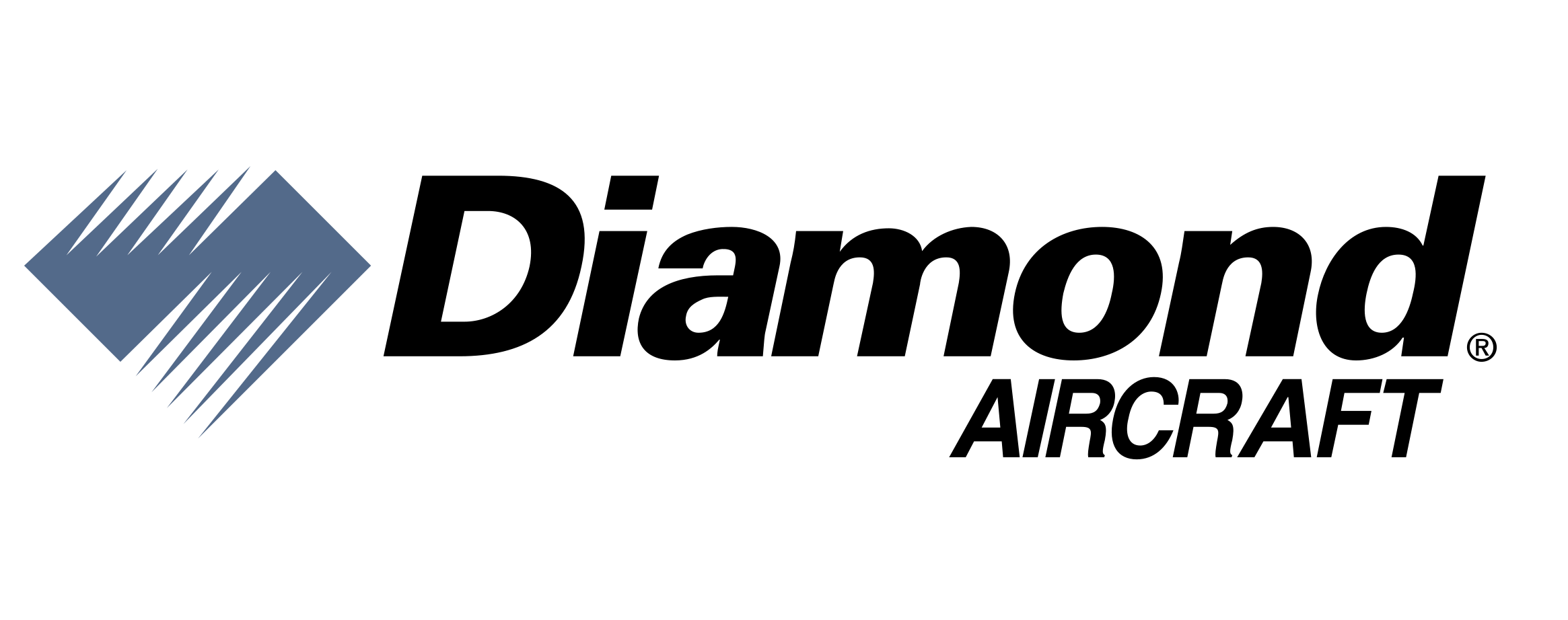
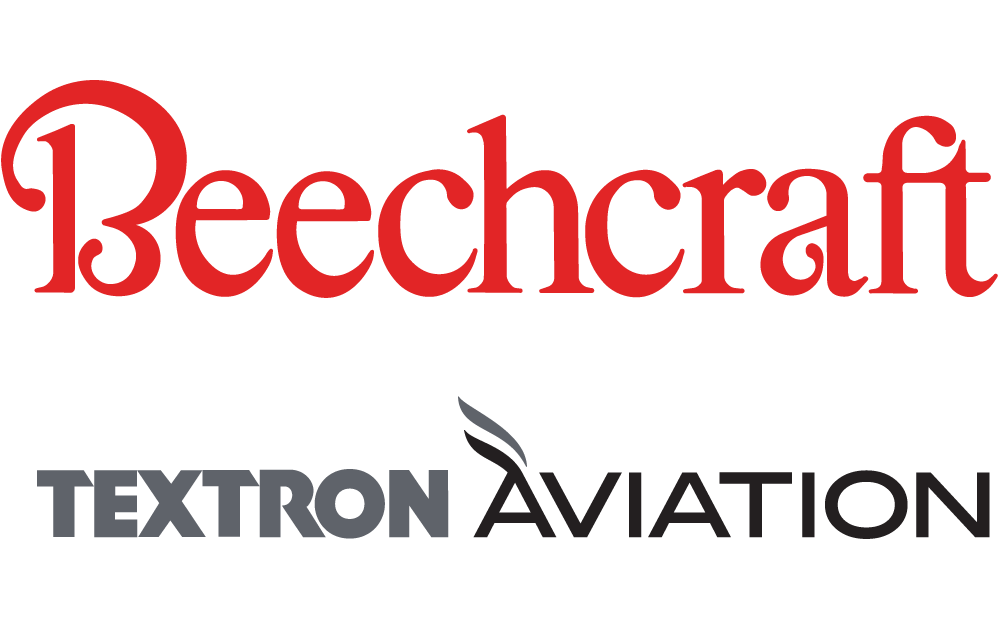
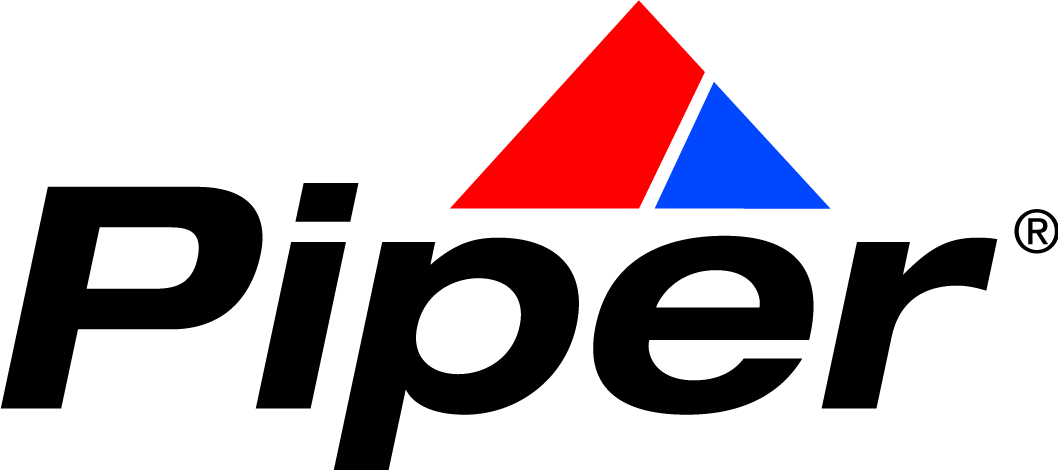
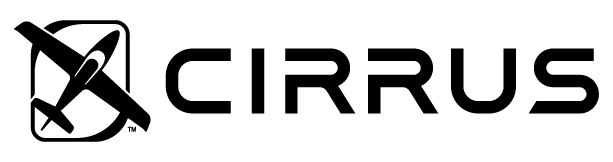